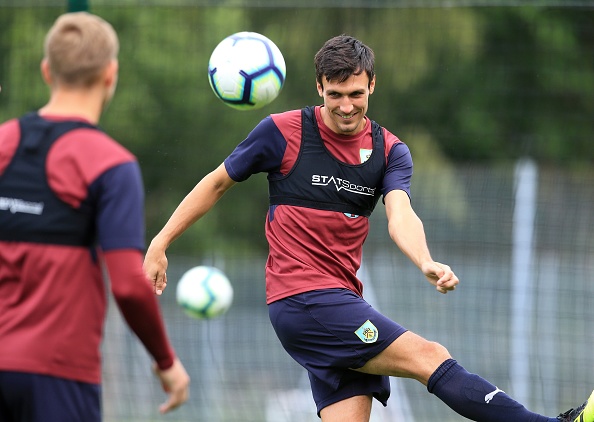
03 Mar A Multi-Mechanical Model Approach to Training Load Monitoring
Introduction
Elite team sport athletes are exposed to a wide range of daily and weekly demands both on and off the field such as training, competitions and media.
Of these demands, training is a significant and essential activity to maximise an athlete’s athletic performance.
It is therefore essential that training is prescribed appropriately in order to avoid overtraining and/or injury.
Monitoring training load allows practitioners to determine whether players are adapting to their training programs, understanding an individual’s response to training, assessing fatigue and the associated need for recovery, whilst minimising the risk of non-functional overreaching, injury, and illness [1].
In recent years with the evolution and development of Global Positioning Systems (GPS), practitioners now have the means to monitor the external load of their athletes during both games and training sessions.
Having access to this information may have a positive impact when reducing the number of injures within a season, with a relationship being identified between running loads and lower body soft tissue injuries [2].
It is therefore important as a practitioner to be able to relay this information to relevant coaching staff in order to plan and periodise training accordingly.
Multi-Mechanical Models
Training load metrics have been typically analysed and reported in isolation without association to training outcomes. E.g. Player X total running distance for today’s session was 6000 metres with 300 metres of that being at high intensity.
Although this can be important information in understanding the session, it can be difficult in some cases for practitioners to provide context to coaches and players on what the values really mean in relation to their game demands.
This is where the concept of a multi-mechanical model approach has been developed, to analyse external loads of training whilst allowing data to be presented to coaches/players in a form that can offer them more context to the games they play.
Essentially what a multi-mechanical model offers is the ability to summarise a session or drill into a volume and intensity score which is based on a percentage of the player’s individual match demands.
To create the score for each player a simple equation is required [Figure 1]. This involves a combination of key metrics, dividing each metric value by what is done in a game for the individual player and then multiplied by 100 to create a percentage score.
Figure 1: Volume/Intensity equation (Owen et al., 2017)
When deciding what key metrics to include within your volume and intensity equation, it’s important to discuss what is relevant for you as a practitioner and as a club.
How the team plays should be a factor in determining what metrics are used by utilising key metrics related to team performance.
It’s also important to take into consideration metrics associated with high potential risk factors for players, such as soft tissue injuries from high total distance and high speed running values.
Accelerations and decelerations are also common metrics to include within the model due to the high mechanical stress and biomechanical load they have on a player’s body.
Once metrics are decided on, match data needs to be recorded and used within the equation. This can either be in the form of max outputs for each metric or match day averages, with the latter offering a better overview of a player’s typical match day output.
Practical Considerations
Although research is very limited in the validity of this model, it does offer a simpler way to report training load data whilst giving added context in relation to a player’s match day demands.
The model has been shown to have significant training load variations in both key session volume and session intensity scores when assessed across a typical microcycle [Figure 2].
Figure 2: Volume/Intensity equation example
The model also concurs with traditional periodisation/tapering models using single metric methods in reporting training outcomes, which report how training load should vary in order to facilitate optimal physiological adaptation [3,4].
With the release of the new software platform ‘Sonra’ by STATSports, practitioners now have the necessary functionality available to utilise this model within the software itself.
The new Custom Metric Calculator allows the user to create their own bespoke volume and intensity metrics and include them within their customisable dashboards and reports [Figure 3].
Figure 3: Total Volume/Intensity across training microcycle (Owen et al., 2017)
This allows the user to easily split sessions and drills into percentage volume and intensity scores compared to a game.
The information can then be provided to allow extra context to a coach or player and enable the practitioner to build a better understanding of the outputs of each drill that has been performed.
It has been said that you should train how you play and by having this information you can easily identify if players are reaching match day intensities within specific drills.
It can also be used within other types of training such as return to play protocols to identify whether a player is hitting the required volume/intensity percentage within sessions or specific drills.
Figure 4: Total Volume/Intensity within Sonra
Conclusion
It is evident that a multi-mechanical approach to training load monitoring can have a positive impact in the day to day reporting to coaches and players.
Having the ability to summarise sessions and drills into simple volume and intensity scores allows the practitioner to add context in relation to player’s match day demands.
However, due to the limited research into the validity of such a concept it is recommended by this sport scientist that practitioners should consider the approach of incorporating both a typical report in isolation as well as utilising a volume and intensity score within your reports.
This will add context to your data and help improve communication of training loads between performance staff, players and coaches.
References
- Bourdon, P.C., Cardinale, M., Murray, A., Gastin, P., Kellmann, M., Varley, M.C., Gabbett, T.J., Coutts, A.J., Burgess, D.J., Gregson, W. and Cable, N.T., 2017. Monitoring athlete training loads: consensus statement. International journal of sports physiology and performance, 12(s2), pp.S2-161.
- Gabbett, T.J. and Ullah, S., 2012. Relationship between running loads and soft-tissue injury in elite team sport athletes. The Journal of Strength & Conditioning Research, 26(4), pp.953-960.
- Owen, Adam L., Leo Djaoui, Matthew Newton, Shane Malone, and Bruno Mendes. “A contemporary multi-modal mechanical approach to training monitoring in elite professional soccer.” Science and medicine in football1, no. 3 (2017): 216-221.
- Malone, J.J., Di Michele, R., Morgans, R., Burgess, D., Morton, J.P. and Drust, B., 2015. Seasonal training-load quantification in elite English premier league soccer players. International journal of sports physiology and performance, 10(4), pp.489-497.
Author Details
Jack McCreanor
Sport Scientist l Sport Science Dept.